‘You’ve got to be data-driven’: the fashion forecasters using AI to predict the next trend
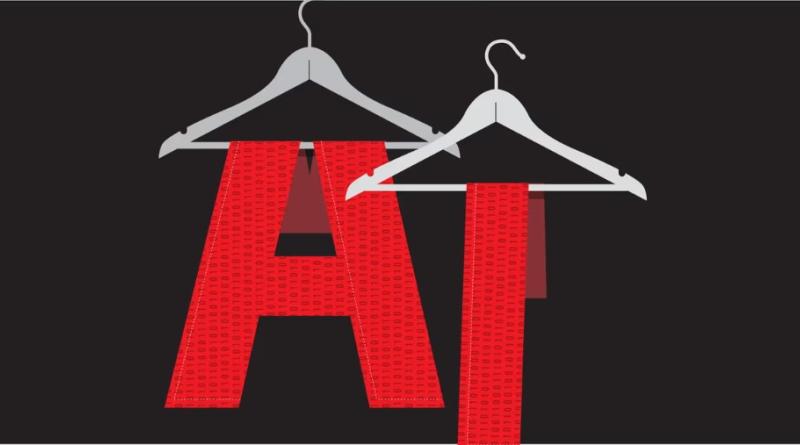
Artificial intelligence can help predict style crazes, shape collections and help the environment by cutting waste material
It’s Paris fashion week and the streets of the city are filled with celebrities, designers, models and journalists. Among the crowds, eagle-eyed experts are taking careful notes. These are the fashion industry’s trend forecasters. Their job is to get a sense of the colours, cuts, fabrics and patterns in the designers’ new collections, in the hope of detecting emerging trends.
Their notes will quickly be added to curated “trend forecasts”, which will be sold to designers and high street retailers, who will use them to inspire new pieces and decide what to stock next season – think of the “blue sweater” speech in The Devil Wears Prada, where Meryl Streep’s character scathingly explains this process to her naive assistant Andy (played by Anne Hathaway). Traditionally, fashion forecasters have relied solely on these qualitative methods, observing runway shows, alongside street fashion and pop culture, to make predictions.
But as artificial intelligence technologies have become increasingly powerful in the last decade, forecasting agencies are now turning to quantitative results generated by machine learning for help. These AI tools can detect patterns within large datasets of runway show images, social media posts, search data, and online and in-store sales data – which can help forecasters spot emerging trends more accurately and, crucially, more quickly.
This is all welcome news for an industry worth about $2tn (£1.65tn) globally, as being even slightly off the mark in colour palettes or hemline decisions can have significant consequences for a company’s bottom line.
Forecasts can also have big implications for the fashion industry’s carbon footprint, which is estimated by the Global Fashion Agenda and McKinsey & Co to be responsible for at least 4% of all worldwide emissions and which chucks more than 92m tonnes of fabric away every year.
“Accurate trend forecasts can reduce waste, save money and give companies an edge over their competitors,” says Dr Michal Koren, a researcher who specialises in fashion forecasting at Shenkar College in Tel Aviv, Israel. “Using AI tools in forecasting can contribute to making the fashion industry more sustainable by reducing the likelihood of inaccurate predictions.”
While it’s worth remembering that AI comes with its own environmental costs, the direct incentive for using these AI forecasting tools is clear: the more accurate the forecasts, the less likely a company will be to waste resources producing clothes no one wants to buy.
“To constantly improve our trend forecasting and only produce what we can sell is an important piece of the puzzle to reach our goal of becoming net zero by 2040,” says Lise-lotte Löveborg, who leads the fashion intelligence team at H&M’s headquarters in Stockholm. “To that end, we are starting to explore how AI can play a part in our forecasting process.”
Edgy, trendy or mainstream
Most industries use forecasting methods in some capacity to decide what, and how much, to produce each year. Usually, these forecasts are based on historical sales data, while adjusting for basic factors such as seasonality (for example, snow tyres tend to sell more in the winter, ice-cream is a safe bet in the summer).
But forecasting in the fashion industry can be trickier. For one thing, factors that are harder to measure, such as emotions and aesthetics, can have a much bigger effect on fashion trends than they do on, say, car tyre sales.
Social and cultural movements can also massively influence trends – think how hot pink invaded the market during this summer’s Barbie movie release, or how bizarre fashion became in the wake of the Covid lockdowns.
“The fast pace of the fashion industry also means trends can change overnight,” says Koren. “For example, if a celebrity just had a sold-out concert, and they happen to be wearing a specific pair of shoes on stage, that can have a dramatic effect on the sales of these products.” Novelty is also key, which poses an innate problem for forecasters. Trends are constantly evolving – as designer Karl Lagerfeld once said: “The essence of fashion is changeability” – which means that what was in vogue in June may have worn itself out by October.
All these factors make it important for fashion forecasters to react quickly to avoid offering their clients outdated predictions. AI tools can be particularly helpful in this regard: AI models are being developed that can automatically scan thousands of runway images and instantly extract the most prevalent colour palettes and patterns.
Forecasters are also looking to big data gathered from social media posts to pull out consumer preferences, as some Instagram influencers now have at least as much trendsetting power as big-name designers – if not more. For instance, AI models such as those developed by the Paris-based company Heuritech analyse millions of images posted on social media each day. They scan posts related to clothes and fashion, classifying users as either “edgy” (the people wearing novel styles that may or may not reach the mainstream, but which are key signals of upcoming trends), “trendy” (the influential early adopters of a trend who will help propagate it), or “mainstream” (the rest of us).
These are then coded from a list of 2,000 attributes – “pleated skirt”, “scarlet colour”, or “long sleeves”, for example – and tracked in real time to allow the AI model to determine how the popularity of specific looks is evolving.
“The goal is to help brands to quantify what people are currently wearing and to be able to predict what people will wear in the future, thanks to all the data out there,” says Tony Pinville, who co-founded Heuritech in 2013 after completing his PhD in artificial intelligence and machine learning at the Sorbonne University in Paris.
Predictions can be generated up to one year in advance and results can be as specific as: “Pinstripes are forecasted to be 80% more visible in the European market in the next year” (that’s a real prediction from Heuritech’s model, by the way, if you are looking for style tips).
As these AI models become increasingly accurate, the hope is that designers and retailers will produce more of what will actually sell. “One of our aims is to tackle the overstock problem in the fashion industry,” says Pinville. “Because at the moment, 40% of fashion products worldwide are not sold at full price and 25% of the products are not sold at all.”
AI tools may also help diversify collections by pulling out trend patterns from demographics that forecasters may otherwise have overlooked. “For example, often we see that designers in France have a very ‘Parisian’ point of view in the fashion industry and they assume their customers have these tastes too,” says Pinville. “But if you look at the data more globally, you can see that the customer segmentation is actually very broad.”
In other words, using AI tools that can analyse millions of posts from around the world each day allows forecasters to see how trends are evolving across specific regions, age groups and subcultures. This gives designers and retailers valuable information when deciding how to adjust their design and stock decisions for each individual market.
Creative angle
But in an emotions-driven industry such as fashion, there are risks to relying solely on the data. “A few years ago, there were big indicators on social media of yellow being a trend,” says Francesca Muston, vice-president for fashion at WGSN, a leading trend forecasting company based in London, which developed its own in-house AI model to incorporate into its prediction methods in 2022. “And if you had followed purely the social media data, yellow pieces would seem to be a good investment, since these posts got loads of likes and shares – probably because people tend to have a very visceral reaction to bright colours.
“But as an individual, are you going to go out and wear a bright yellow coat yourself? That’s a brave fashion choice. Yet the people who are going out in black and grey coats may well have liked those yellow coat posts on social media.”
That’s where the traditional skills of a trend forecaster remain key. By combining large-scale data results with creative expertise and an understanding of the wider social context, forecasters can usefully interpret the data to create predictions that will be relevant to the average consumer.
“That’s why we maintain a very strong creative angle in our forecasting, with a team of industry experts,” says Muston. “So if we’re forecasting, for example, for the beauty industry, that team of people may have worked on formulations of face creams and will have an innate understanding of the product.”
WGSN’s methodology also relies on what it calls “foundational research” to create more comprehensive forecasts, accounting for qualitative elements such as how changes in government legislation or youth culture may influence trends. During the 2020 Covid pandemic, this included monitoring how, for example, government-imposed lockdowns would affect things such as loungewear sales (which rocketed) or wedding dress revenues (which plummeted).
Retaining these qualitative elements is important, since AI tools are only able to aggregate existing information about what people are wearing and make predictions accordingly. They also don’t have their own aesthetic perspective or fashion sense – at least not yet – so they may not be able to satisfy consumers’ desire for newness, which is often an integral part of what makes something trendy.
“The role of human experts in interpreting and curating trends will continue to be crucial even as AI and data-driven models become more accurate,” says Koren. This is because, ultimately, trend forecasters must also have a broader sense for reading the cultural and political vibe – and understanding how this will translate into clothing.
“For instance, in 2008, the fashion industry was significantly impacted by the global financial crisis and forecasters understood that for the new summer clothes to be relevant, they had to reflect the dismal economic situation,” Koren explains. “That’s why clothes of that season were described as ‘depression chic’ and why black dominated the [autumn] 2009 colour palette.”
So it seems, for the moment, AI forecasting tools are just that – a tool that can be used alongside others to make more informed creative decisions. “To be a good trend forecaster these days, you’ve got to be both data-driven and creatively led,” says Muston. “Because at the end of the day, it’s about interpreting all of the signals out there to map out the future.”
Illustration: Paul Tansley/Observer Design